Unfolding the Significance of Sampling in Research Methodology: An In-Depth Exploration
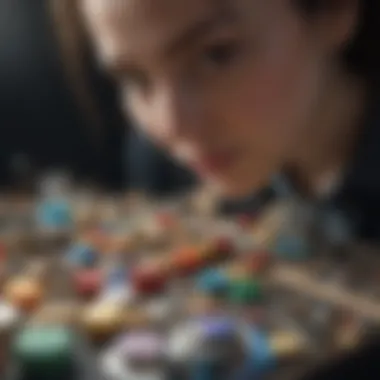
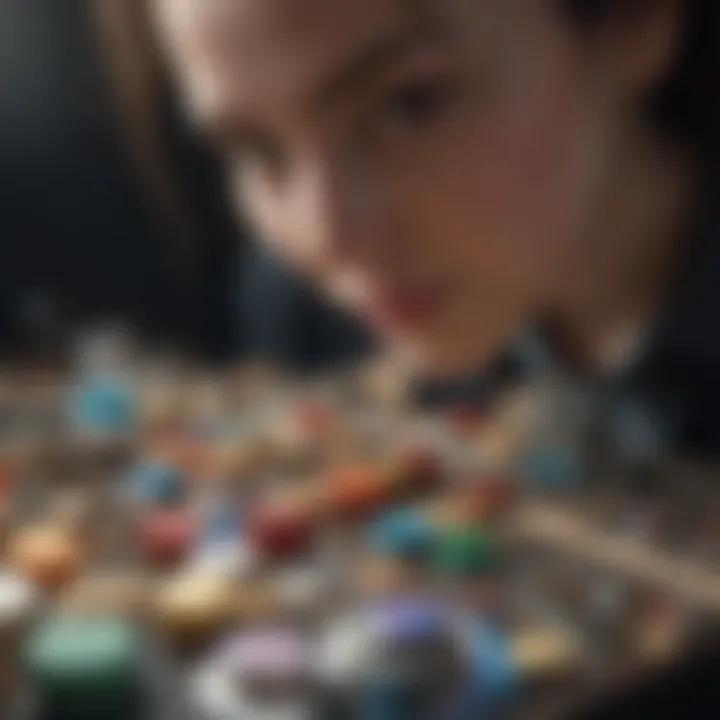
Understanding the Significance of Sampling in Research Methodology
Sampling plays a pivotal role in research methodology, underpinning the reliability and validity of study outcomes. By selecting a representative subset of the population, researchers can draw conclusions that generalize to the broader target group. Understanding the nuances of different sampling methods is imperative for ensuring the quality and credibility of research findings.
What is Sampling in Research Methodology?
Sampling refers to the process of selecting a subset of individuals or elements from a larger population to study and draw inferences. It involves carefully choosing sample members to minimize bias and ensure the findings are applicable to the entire population.
The Fundamental Role of Sampling
Sampling is the bedrock of research methodology, shaping the accuracy and applicability of study results. Whether employing random sampling, stratified sampling, convenience sampling, or other techniques, researchers must adhere to methodological rigor to enhance the robustness of their conclusions.
Why Valid Sampling Matters
Valid sampling is essential for obtaining reliable research outcomes. A well-executed sampling strategy increases the likelihood of producing results that reflect the true characteristics of the entire population, bolstering the study's credibility and relevance across various disciplines.
The Influence of Sampling Methods on Research Outcomes
In the landscape of research, the selection of sampling methods exerts a profound impact on study outcomes and the subsequent interpretations of data. Different sampling approaches, such as probability and non-probability sampling, have distinct implications for research design, sampling adequacy, and result generalizability. Researchers must navigate these methodological choices thoughtfully to ensure the robustness and validity of their research endeavors.
Introduction:
In the realm of research methodology, sampling holds paramount importance as it serves as the cornerstone of robust and reliable study outcomes. An intricate understanding of various sampling methods and techniques is imperative across a multitude of disciplines to ensure the integrity and validity of research findings. Driven by the fundamental premise of selecting a subset from a larger population, sampling practices dictate the efficacy of research endeavors.
Defining Sampling in Research:
Understanding the Concept of Sampling:
Sampling encompasses the process of selecting a representative sample from a larger population to draw inferences and make generalizations. The inherent significance lies in the ability to extrapolate findings from the sample to the population itself, thereby enhancing the credibility and applicability of research outcomes. This methodological approach is characterized by its ability to provide a snapshot of the larger population while mitigating the time and resource constraints often associated with exhaustive data collection. Despite its widespread adoption, challenges such as sampling bias and inadequate sample size may pose limitations to the efficacy of this approach.
Importance of Sampling in Research:
Sampling plays a pivotal role in research by diminishing the complexities associated with studying entire populations. By strategically selecting a subset that faithfully represents the population characteristics of interest, researchers can extrapolate findings with heightened accuracy and efficiency. This ensures that research outcomes are both reliable and actionable, bolstering the credibility and utility of the study results. However, the meticulous design and execution of sampling strategies are critical to circumvent potential biases and confounding variables that may compromise the integrity of the research findings.
Link Between Sampling and Generalizability:
The link between sampling methodology and generalizability is intrinsic to the research process, underpinning the extent to which study results can be extrapolated to a broader population. A well-executed sampling strategy enables researchers to draw inferential conclusions that hold true beyond the confines of the sample group, thereby enhancing the external validity of the study. However, considerations such as sample representativeness and scale must be diligently addressed to ensure that generalizations derived from the sample align with the characteristics of the entire population.
Types of Sampling:
Probability Sampling:
Probability sampling methods entail a systematic approach to sample selection that affords every unit in the population an equal chance of being included in the sample. This methodology adheres to the principles of randomness and ensures that each sample subset is a true reflection of the larger population. While conducive to generalizability and statistical inference, probability sampling requires meticulous planning and execution to mitigate biases stemming from non-response or sampling errors.
Non-Probability Sampling:
Unlike probability sampling, non-probability sampling methods are founded on non-random selection techniques that do not guarantee equal representation of the population. This approach is often employed when logistical constraints or specialized research objectives necessitate targeted sampling. Despite its utility in certain research contexts, non-probability sampling is susceptible to selection bias and may compromise the generalizability of study findings.
Stratified Sampling:
Stratified sampling involves dividing the population into distinct subgroups based on predetermined criteria, with samples then being randomly selected from each stratum. This method enables researchers to account for variability within the population and ensure proportional representation of key demographic or characteristic factors. By enhancing the precision of estimates and comparisons across subgroups, stratified sampling amplifies the robustness of research outcomes while reducing sampling errors inherent in other sampling methods.
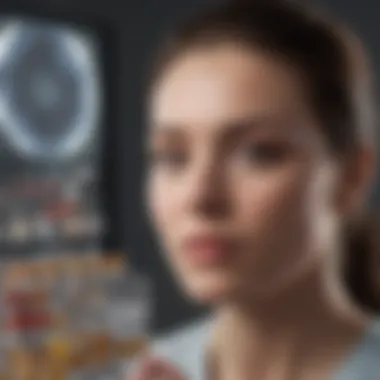
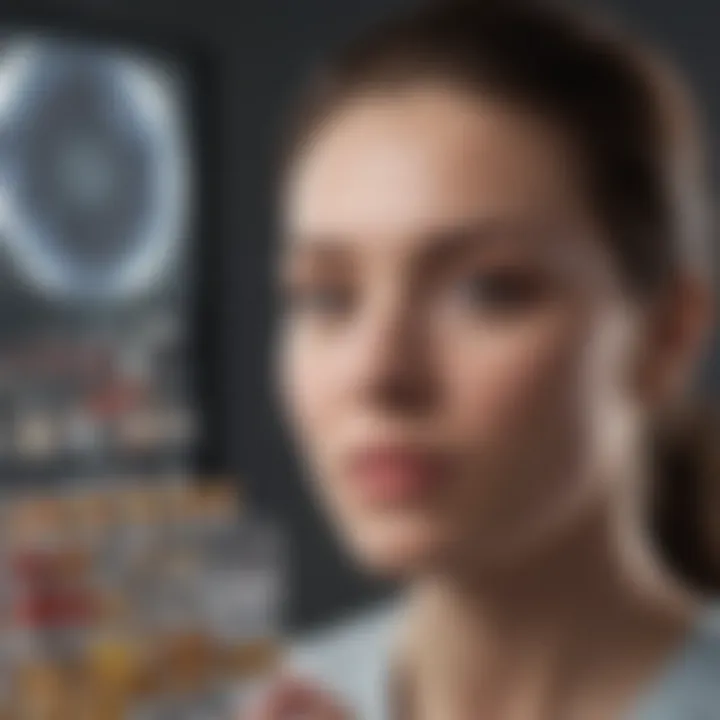
Cluster Sampling:
Cluster sampling revolves around the division of a population into clusters or groups, with entire clusters being selected for inclusion in the sample. This approach streamlines data collection in geographically dispersed populations or clustered settings, optimizing research efficiency while accommodating logistical constraints. However, cluster sampling may introduce clustering effects and increase the potential for sample homogeneity, necessitating careful consideration of cluster size and structure to uphold the integrity of the research findings.
Convenience Sampling:
Convenience sampling entails the selection of readily accessible participants based on convenience or proximity to the researcher. While this approach expedites data collection and simplifies participant recruitment, it may introduce selection bias and compromise the external validity of the study. Researchers must exercise caution when employing convenience sampling to ensure that sample characteristics align with the research objectives and target population.
Purposive Sampling:
Purposive sampling involves the intentional selection of participants who possess specific characteristics or attributes that align with the research objectives. This method enables researchers to target individuals who can provide unique insights or specialized knowledge, enhancing the depth and relevance of the study findings. Despite its value in certain research contexts, purposive sampling demands a comprehensive understanding of the target population and research objectives to ensure the strategic selection of participants.
Snowball Sampling:
Snowball sampling utilizes existing participants to recruit additional individuals who meet the study criteria, creating a chain referral network that expands the sample size. This iterative process is particularly useful in researching hard-to-reach populations or marginalized groups where traditional sampling methods may prove challenging. While snowball sampling facilitates participant recruitment and fosters community involvement, it may introduce sampling bias and limit the generalizability of study findings due to the non-random nature of participant selection.
Sampling Techniques:
Simple Random Sampling:
Simple random sampling involves the random selection of individuals from the population, where each member has an equal probability of being chosen. This method eliminates bias in sample selection and simplifies statistical analysis, facilitating generalizability and inferential conclusions. However, careful attention must be paid to ensuring true randomness in the selection process to uphold the validity and reliability of research outcomes.
Systematic Sampling:
Systematic sampling employs a predetermined interval to select sample units at regular intervals from the population list, offering a systematic yet simplified approach to sampling. By establishing a structured sampling framework, researchers can streamline data collection and mitigate biases arising from irregular patterns or clustering within the population. However, the effectiveness of systematic sampling hinges on the adequacy of the sampling interval and the absence of periodicity in the target population.
Quota Sampling:
Quota sampling involves the intentional selection of individuals based on pre-defined quotas or proportions to mirror specific population characteristics. This method enables researchers to ensure adequate representation of key demographic variables within the sample, enhancing the external validity and comparability of study results. While quota sampling expedites participant recruitment and enhances sample diversity, researchers must exercise caution to prevent sampling bias and uphold the integrity of the research outcomes.
Multi-Stage Sampling:
Multi-stage sampling encompasses a multi-tiered approach to sample selection, where sampling occurs in multiple stages or phases. This method enables researchers to hierarchically sample from larger to smaller units, accommodating complex population structures or nested sampling frameworks. By systematically capturing variance across different levels of sampling, multi-stage sampling enhances the precision and representativeness of research findings while minimizing resource-intensive data collection processes.
Factors Influencing Sampling Decisions
Sampling decisions play a vital role in the realm of research methodology, shaping the very foundation upon which studies are conducted. Understanding the nuances of factors that influence sampling decisions is imperative for researchers aiming for robust and credible results. Key elements to consider include the characteristics of the population under study, the specific research objectives, and the constraints posed by available resources.
Population Characteristics
Homogeneity vs. Heterogeneity
In the context of research, the distinction between homogeneity and heterogeneity within a population holds immense significance. Homogeneity refers to a scenario where the individuals in a population share similar characteristics, while heterogeneity denotes diversity among the population members. Opting for a homogeneous population can enhance the precision of results in certain types of studies where consistent trends are sought. However, heterogeneous populations offer broader insights into real-world complexities, potentially enriching the study's applicability.
Size of Population
The size of the population being studied is a crucial consideration influencing sampling decisions. A large population may increase the representativeness of the sample but also demands more resources for data collection and analysis. Conversely, a small population size might limit generalizability but could provide in-depth insights into specific phenomena. Finding the right balance between sample size and population characteristics is key to the success of a research endeavor.
Accessibility of Population
The accessibility of the population under study impacts the feasibility and practicality of sampling methods. Easy access to the population can streamline data collection processes, reducing time and costs. However, challenges may arise when dealing with populations that are geographically dispersed or harder to reach. Researchers must weigh the trade-offs between convenience and representativeness when considering the accessibility of the population.
Research Objectives
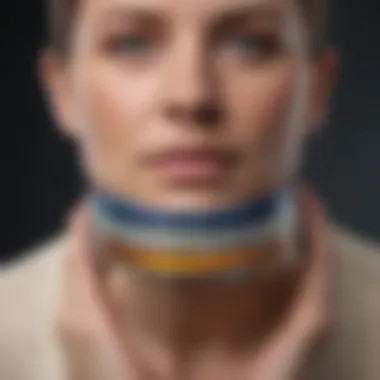
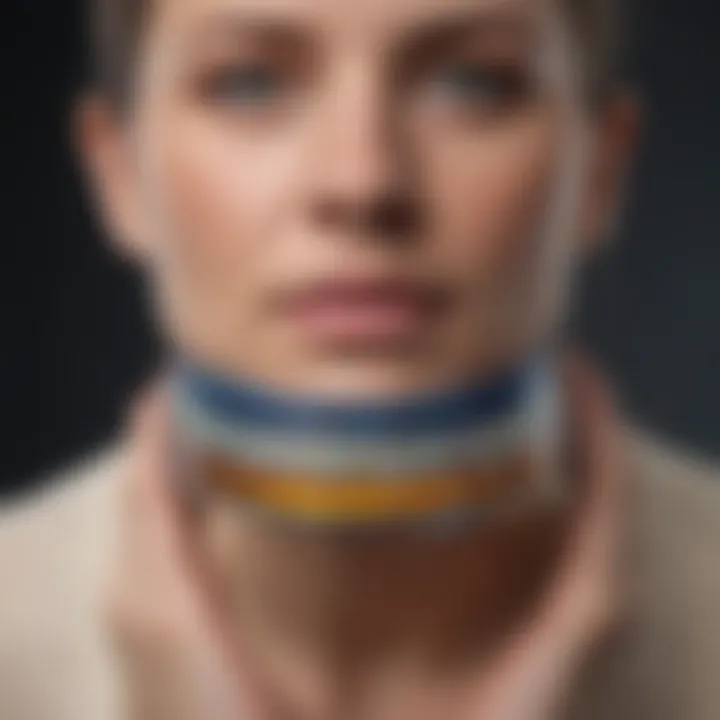
Exploratory Studies
Exploratory studies aim to delve into uncharted territory, seeking to generate hypotheses and explore new phenomena. By embracing open-ended inquiries and allowing for flexibility in data collection, exploratory studies offer valuable insights that can guide future research endeavors. However, the less structured nature of exploratory studies may pose challenges in result interpretation and generalizability.
Descriptive Studies
Descriptive studies focus on portraying the characteristics of a population or phenomenon under investigation. The goal is to provide a comprehensive snapshot of the subject matter, often through surveys, observations, or content analysis. Descriptive studies facilitate the understanding of prevalence, distribution, and associations within the study context, laying the groundwork for further research. Yet, limitations may arise concerning the depth of analysis and the establishment of causal relationships.
Causal Studies
Causal studies delve into establishing cause-and-effect relationships between variables, aiming to uncover the mechanisms driving observed phenomena. By employing experimental designs or longitudinal studies, causal studies strive to identify the underlying factors that influence outcomes. While offering conclusive insights, causal studies require meticulous planning and control to mitigate confounding variables and ensure the validity of causal inferences.
Resource Constraints
Time
The element of time plays a critical role in sampling decisions, as research projects often operate within defined timelines. Efficient time management is essential for collecting data, analyzing results, and drawing meaningful conclusions. Limited time resources may restrict the scope of the study or necessitate prioritization of specific research objectives to ensure timely completion.
Budget
Budgetary constraints can profoundly impact the sampling strategies adopted in a research project. Adequate funding is essential for recruiting participants, acquiring materials, and compensating researchers for their efforts. Striking a balance between cost-effectiveness and data quality is imperative, as budget constraints may influence sample size, data collection methods, and data processing techniques.
Manpower
The availability and expertise of research personnel, or manpower, shape the execution of sampling strategies and data collection processes. Adequate manpower ensures the smooth implementation of research protocols, data management, and analysis procedures. However, limited manpower can pose challenges in terms of workload distribution, training requirements, and research output. Efficient utilization of available human resources is crucial for addressing sampling decisions within the constraints of manpower.
Sampling Errors and Mitigation
Sampling errors are a critical consideration in research methodology as they can introduce biases and inaccuracies in study findings. It is vital to understand and address these errors to ensure the reliability and validity of research outcomes. By acknowledging and mitigating sampling errors, researchers can enhance the credibility of their studies and make informed decisions based on accurate data. This section will delve into the various types of sampling errors and strategies to minimize their impact on research results.
Types of Sampling Errors
Random Sampling Error
Random sampling error is a fundamental aspect that researchers must account for in their studies. This error occurs due to the natural variability that can arise when using a sample to estimate parameters of a population. Despite efforts to obtain a representative sample, random sampling error is inevitable to some degree. However, understanding the distribution and potential impact of random sampling error can help researchers interpret their findings with caution, considering the margin of error associated with their estimates and recommendations.
Systematic Sampling Error
Systematic sampling error, unlike random error, results from consistent and repeatable mistakes in the sampling process. This type of error can skew results in a particular direction, leading to biased conclusions. Researchers need to identify and address systematic errors through diligent review of sampling methods and data collection procedures to ensure the integrity of their study outcomes. By minimizing systematic errors, researchers can enhance the accuracy and validity of their research findings.
Non-Response Error
Non-response error arises when selected participants fail to provide data or participate in the study, leading to incomplete information and potential bias in the results. Researchers must address non-response error by exploring reasons for participant non-compliance and implementing measures to encourage higher response rates. By addressing non-response error effectively, researchers can improve the quality and representativeness of their research data, resulting in more reliable conclusions and actionable insights.
Strategies to Address Sampling Errors
Increasing Sample Size
Increasing the sample size is a common strategy employed to reduce the impact of sampling errors, particularly random error. By including more participants in the study, researchers can improve the precision of their estimates and reduce the margin of error associated with their findings. However, expanding the sample size also entails additional costs and resources, necessitating a careful balance between statistical power and practical constraints.
Improving Sampling Techniques
Enhancing sampling techniques involves refining the methods used to select participants and collect data to minimize errors and biases. By adopting rigorous and systematic sampling approaches, researchers can enhance the representativeness and reliability of their study results. Improved sampling techniques contribute to the credibility of research findings and support robust conclusions based on sound methodological practices.
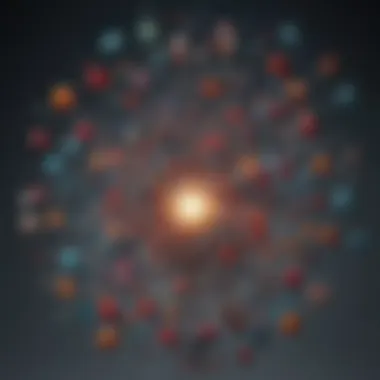
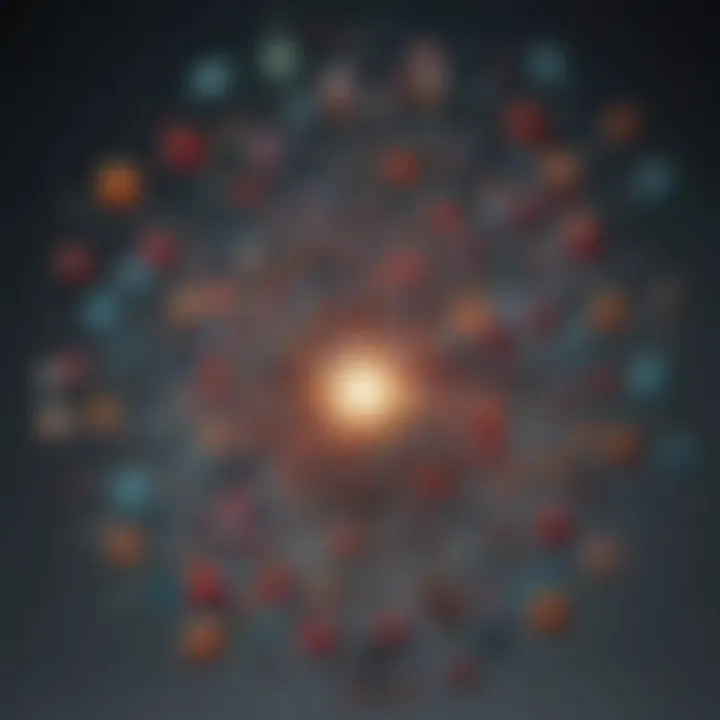
Reducing Non-Response Rates
Reducing non-response rates is crucial in mitigating the impact of incomplete data on research outcomes. Researchers can address non-response by employing effective communication strategies, follow-up protocols, and incentives to encourage participant engagement. By decreasing non-response rates, researchers can enhance the completeness and accuracy of their data, improving the robustness of their study conclusions and enhancing the overall quality of their research outcomes.
Ethical Considerations in Sampling
In the realm of research methodology, an area of paramount importance is the ethical considerations in sampling. Ethical considerations play a crucial role in ensuring the integrity and credibility of research outcomes. By prioritizing ethical practices in sampling, researchers uphold the rights and well-being of participants, thus fostering trust and respect within the academic community. The ethical guidelines provide a framework for maintaining the dignity and autonomy of individuals involved in research studies. Ethical considerations in sampling also serve to protect vulnerable populations and prevent any potential harm or exploitation.
Informed Consent
Protection of Participants' Rights
Discussing the aspect of protection of participants' rights within the realm of ethical considerations is pivotal. Protecting participants' rights involves safeguarding their autonomy, privacy, and confidentiality throughout the research process. By obtaining informed consent from participants, researchers acknowledge and respect individuals' right to make voluntary and informed decisions regarding their involvement in a study. This practice enhances the transparency and trustworthiness of the research endeavor, contributing to the overall ethical integrity of the study.
Protection of participants' rights is a cornerstone of ethical research practices, ensuring that individuals are treated with dignity and respect. Respecting participants' rights not only upholds ethical standards but also builds a foundation of trust between researchers and participants. However, it is essential to recognize that balancing participants' rights with the goals of the study can sometimes present challenges, necessitating careful navigation to maintain ethical standards.
Confidentiality and Anonymity
Another critical aspect of ethical considerations in sampling is ensuring confidentiality and anonymity for participants. Maintaining confidentiality involves safeguarding participants' personal information and research data from unauthorized access or disclosure. Anonymity, on the other hand, entails protecting individuals' identities by using pseudonyms or other identifiers to conceal their identities in research reports.
Confidentiality and anonymity are essential components of ethical research practices, as they help mitigate risks related to privacy breaches and ensure the protection of participants' sensitive data. By guaranteeing confidentiality and anonymity, researchers demonstrate their commitment to upholding ethical standards and preserving participants' trust. Nevertheless, maintaining confidentiality and anonymity may pose challenges in certain research contexts, influencing the study's design and implementation.
Avoiding Bias and Manipulation
Ensuring Fair Selection Processes
The imperative of ensuring fair selection processes emerges as a fundamental element in ethical research practices. Fair selection processes involve unbiased and transparent methods for recruiting participants, aiming to prevent any form of discrimination or favoritism. By adopting fair selection processes, researchers uphold the principles of equity and inclusivity in participant recruitment, contributing to the overall validity and reliability of the study.
Ensuring fair selection processes is indispensable for fostering trust and credibility in research outcomes. By adhering to fair selection practices, researchers mitigate the risk of bias and promote the representation of diverse perspectives within the study cohort. However, implementing fair selection processes requires careful planning and implementation to address potential challenges and minimize the influence of external factors.
Avoiding Coercion or Undue Influence
In the domain of ethical considerations, avoiding coercion or undue influence represents a vital mandate for maintaining the ethical integrity of research practices. Coercion refers to the use of force or pressure to compel individuals to participate in a study against their will, while undue influence involves the offering of excessive incentives that may compromise participants' autonomy or decision-making capacity.
Avoiding coercion or undue influence is paramount for respecting participants' autonomy and ensuring their voluntary participation in research activities. By refraining from coercive tactics and undue inducements, researchers uphold the principles of autonomy and beneficence, safeguarding participants' well-being and rights. However, navigating the complexities of avoiding coercion or undue influence demands ethical sensitivity and vigilance to uphold the ethical standards of research conduct.
Best Practices in Sampling
In the realm of research methodology, the concept of best practices in sampling holds a paramount position in ensuring the credibility and robustness of the study outcomes. Delving into this subject matter within the contours of this article becomes imperative to grasp the intricate nuances that dictate the quality of research findings. By shedding light on the specific elements, benefits, and considerations tied to best practices in sampling, this section aims to provide a comprehensive guide for researchers across diverse domains seeking to enhance the reliability and validity of their studies.
Maintaining Transparency
Clearly Defined Sampling Procedures
The crux of conducting a study that upholds the principles of transparency lies in its sampling procedures. Clearly defined sampling procedures act as the guiding light, steering researchers towards a structured and methodical approach in selecting participants for their studies. The essence of this approach lies in its precision and clarity. It eliminates ambiguities, assuring both researchers and stakeholders of the integrity of the sampling process. This consistency in defining sampling procedures proves to be a pillars against which the study's credibility stands, offering a clear roadmap for data collection and analysis. While the rigid structure of clearly defined sampling procedures ensures standardization and reliability, its rigidity can at times limit the flexibility needed for certain types of research studies in this article.
Documentation of Sampling Methodologies
Ensuring a meticulous documentation of sampling methodologies plays a pivotal role in adding layers of credibility to research endeavors. By articulating the process of sampling methods, researchers not only enhance the reproducibility of their work but also establish a strong foundation of accountability within their study. This documentation not only acts as a roadmap for the research team but also serves as a reference point for future investigations or audits. The virtue of detailed documentation lies in its ability to withstand the test of time, providing a historical perspective on the evolution of sampling techniques used in this article.
Continuous Evaluation and Adaptation
Regular Assessment of Sampling Techniques
A critical aspect that propels research methodologies towards quality outcomes is the regular evaluation of sampling techniques. By periodically scrutinizing and evaluating the efficacy of selected sampling methods, researchers can calibrate their approaches and rectify any discrepancies that may compromise the integrity of their study. The cornerstone of this practice lies in its iterative nature, allowing researchers to fine-tune their sampling strategies based on real-time feedback and insights gained during data collection. The dynamic nature of regular assessment ensures that the research stays aligned with its objectives, enhancing the overall robustness of findings while fulfilling the stringent quality standards in this article.
Flexibility in Sampling Approaches
Embracing a flexible stance towards sampling approaches epitomizes the essence of adaptability in the realm of research methodology. This agility in approach equips researchers with the ability to pivot swiftly in response to unforeseen circumstances or emerging trends within the research landscape. The hallmark of flexibility in sampling approaches lies in its capacity to accommodate changing research dynamics, ensuring that the study remains relevant and effective in meeting its intended goals. While this flexibility fosters innovation and resilience, an over-reliance on adaptability can sometimes blur the boundaries of methodological rigor in this article.
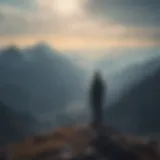
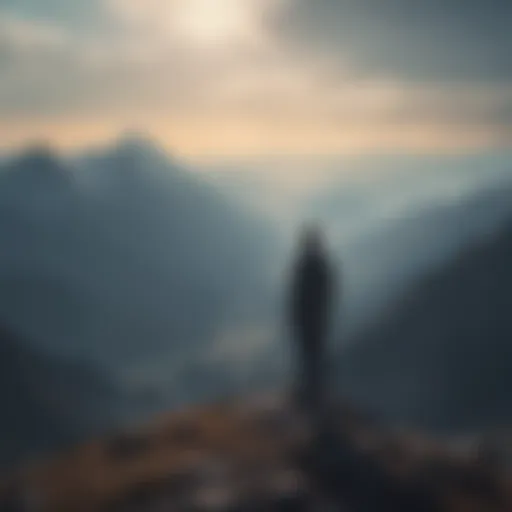